Inteligencia artificial en enfermedades infecciosas
Resumen
Estamos asistiendo a una verdadera revolución tecnológica en el campo de la salud. Los procesos basados en la aplicación de la inteligencia artificial (IA) y el aprendizaje automático (AA) están llegando progresivamente a todas las áreas disciplinares, y su aplicación en el campo de las enfermedades infecciosas es ya vertiginoso, acelerado por la pandemia de COVID-19.
Hoy disponemos de herramientas que no solamente pueden asistir o llevar adelante el proceso de toma de decisiones basadas en guías o algoritmos, sino que también pueden modificar su desempeño a partir de los procesos previamente realizados.
Desde la optimización en la identificación de microorganismos resistentes, la selección de candidatos a participar en ensayos clínicos, la búsqueda de nuevos agentes terapéuticos antimicrobianos, el desarrollo de nuevas vacunas, la predicción de futuras epidemias y pandemias, y el seguimiento clínico de pacientes con enfermedades infecciosas hasta la asignación de recursos en el curso de manejo de un brote son actividades que hoy ya pueden valerse de la inteligencia artificial para obtener un mejor resultado.
El desarrollo de la IA tiene un potencial de aplicación exponencial y sin dudas será uno de los determinantes principales que moldearán la actividad médica del futuro.
Sin embargo, la maduración de esta tecnología es necesaria para su inserción definitiva en las actividades cotidianas del cuidado de la salud requiere la definición de parámetros de referencia, sistemas de validación y lineamientos regulatorios que todavía no existen o son aún sólo incipientes.
Descargas
Citas
Schwartz WB. Medicine and the computer - the promise and problems of change. N Engl J Med 1970; 283:1257-64.
Haug CJ, Drazen JM. Artificial intelligence and machine learning in clinical medicine 2023. N Engl J Med 2023, 388(13):1201-8. doi:10.1056/NEJMra23023038
Lee P, Bubeck S, Petro J. Benefits , limits and risks of GPT-4 as an AI chatbot for medicine. N Engl J Med 2023(18):1233-9
Brownstein JS, Rader B, Astley CM, Tian H. Advances in artificial intelligence for infectious-disease surveillance. N Engl J Med 2023; 388(17):1597-607.
Russell, S. J., Norvig, P. Artificial intelligence: a modern approach 2010. Prentice and Hall, New Jersey, 3a edición, 2019..
Camacho DM, Collins KM, Powers RK, Costello JC, Collins JJ. Next-generation machine learning for biological networks. Cell 2018; June 14, 173:1581-92.
Omrani, H. Predicting travel mode of individuals by machine learning. Transportation research procedia 2015; 10, 840-849.
Ullah, Z., Al-Turjman, F., Mostarda, L., & Gagliardi, R. Applications of artificial intelligence and machine learning in smart cities. Computer Communications 2020, 154, 313-323.
Tripathi, B. K. On the complex domain deep machine learning for face recognition. Applied Intelligence 2017, 47(2), 382-396.
Raju, K., Chinna Rao, B., Saikumar, K., & Lakshman Pratap, N. An Optimal Hybrid Solution to Local and Global Facial Recognition Through Machine Learning. A Fusion of Artificial Intelligence and Internet of Things for Emerging Cyber Systems 2022, 203-226.
Cardona, T., Cudney, E. A., Hoerl, R., & Snyder, J. Data mining and machine learning retention models in higher education. Journal of College Student Retention: Research, Theory & Practice 2020, 1521025120964920.
Tran, H. D., Le, N., & Nguyen, V. H. Customer Churn Prediction in the Banking Sector Using Machine Learning-Based Classification Models. Interdisciplinary Journal of Information, Knowledge, and Management 2023; 18, 087-105.
DeYoung B, Morales M and Giglio S. Microbiology 2.0–A “behind the scenes” consideration for artificial intelligence applications for interpretive culture plate reading in routine diagnostic laboratories. Front. Microbiol 2020; 13:976068. doi: 10.3389/fmicb.2022.976068
Ford, B. A., and McElvania, E. Machine learning takes laboratory automation to the next level. J. Clin. Microbiol 2020; 58:e00012-20. doi: 10.1128/JCM. 00012-20
Van, T. T., Mata, K., and Dien Bard, J. Automated detection of Streptococcus pyogenes pharyngitis by use of colorex strep a CHROMagar and WASPLab artificial intelligence chromogenic detection module software. J. Clin. Microbiol 2019; 57:e00811-19. doi: 10.1128/JCM.00811-19
Glasson, J., Hill, R., Summerford, M., Olden, D., Papadopoulos, F., Young, S., et al. Multicenter Evaluation of an Image Analysis Device (APAS): comparison between digital image and traditional plate reading using urine cultures. Ann. Lab. Med 2017; 37, 499–504. doi: 10.3343/alm.2017.37.6.499
Gammel, N., Ross, T. L., Lewis, S., Olson, M., Henciak, S., Harris, R., et al. Comparison of an automated plate assessment system (APAS independence) and artificial intelligence (AI) to manual plate reading of methicillin-resistant and methicillin-susceptible Staphylococcus aureus CHROMagar surveillance cultures. J. Clin. Microbiol 2021; 59:e0097121. doi: 10.1128/JCM.00971-21
Pascucci M, Royer G, Adamek J, y col. AI-based mobile application to fight antibiotic resistance. Nat Commun 2021;12:1173 https://doi.org/10.1038/s41467-021-21187-3
Human Microbiome Project Consortium. The integrative Human Microbiome Project dynamic analysis of microbiome-host omics profile during periods of human health and diseases. Cell Host Microbe 2014, 16:276-89.
MacIntyre CR, Lim S, Quigley A. Preventing the next pandemic; use of artificial intelligence for epidemic monitoring and alerts. Cell Reports Med 2022, 3, 100867, Dec 20.
Rolland C, Lazarus C, Giese C, Monate B, Travert AS, Salomon J. Early detection of public health emergencies of international concern through Undiagnosed disease reports in ProMED-Mail. Emerg Infect Dis 2020, 26:336-339. https://doi.org/10.3201/eid2602.191043
Bhatia S. Lassmann B, Cohn E, Desai AN, Carrion M, Kraemer MUG, Herringer ;M, Brownstein J, Madoff L, Cori A, Nouvellet P. Using digital surveillance tools for near-time mapping of the risk of infectious diseases spread. NPJ Digit Med 2021, 4,73. https://doi.org/10.1038/s41746-021-00442-3
Brownstein JS, Freifeld CC, Reis BY, Mandl KD. Surveillance Sans Frontières: internet-based emerging infectious disease intelligence and the HealthMap project. PLoS Med 2008:5(7):e151.
Hii A, Chugtai AA, Housen T, Saketa S, Kunasekaran MP, Sulaiman F, Yanti NS, MacIntyre CR. Epidemic intelligence needs stakeholders in the Asia-Pacific region. Western Pac Surveill Response J 2018, 9:28-36. https://doi.org/10.5365/wpsar.2018.9.2.009.
Moa A, Muscatello D, Chughtai A, Chen X, MacIntyre CR. Flucast: a real-time tool to predict severity of an influenza season, JMIR Public Health Surveill 2019, 5, e11780. https://doi.org/10.2196/11780
Lee EWJ, Yee AZH. Toward data sense-making in digital health communication research: why theory matters in the age of big data. Front Commun 2020, 5, doi.10.3389/fcomm.2020.00011.
Laventhal N, Basak R, Dell ML, et al. The ethics of creating a resource allocation and patient triage during the COVID-19 pandemic. Pediatrics 2020, 146(1):e20201243. doi:10.1542/peds.2020-1243.
Thompson RN. Epidemiological models are important tools for guiding COVID-19 interventions. BMC Med 2020, 18(1)152. doi:10.1186/s12916-020-01628-4
Brauner JM, Mindermann S, Sharma M, y col. Inferring the effectiveness of government interventions against COVID-19. Science 2021;371(6531):eabd9338. doi:10.1126/science.abd9338.
Chirino A, Wiemken T, Furmanek S, Mattingly W, Chandler T, Cabral G, Cavallazzi R, Carrico R, Ramirez J. High consistency between recommendations by a pulmonary specialist and ChatGPT for the management of a patient with non- resolving pneumonia. Norton Healthcare Medical Journal. Publicado online Mayo 17, 2023. doi:10.59541/001c.75456
Harrer S, Shah P, Anthony B y col. Artificial intelligence for clinical trial design. Trends Pharmacol Sci 2019, 40(8):577-91. doi:10.1016/j.tips 2019.05.005.
Jain K. Artificial intelligence applications in handling infectious diseases. Primary Health Care 2020, 10 (5):351-353.
Cruz, Y.J.; Rivas, M.; Quiza, R.; Villalonga, A.; Haber, R.E.; Beruvides, G. Ensemble of convolutional neural networks based on an evolutionary algorithm applied to an industrial welding process. Computers in Industry 2021, 133. doi:10.1016/j.compind.2021.103530.
Wang H, Jia S, Li Z, Duan D, Tao G y Zhao Z. A comprehensive review of artificial intelligence in prevention and treatment of COVID-19 pandemic.Front Genet 2022; Vol 13: 845305 doi:103389/fgene.2022.845305
Chowdhury MEH, Rahman T, Khandakar A, Mazhar R, Kadir MA, Mahbub ZB y col. Can AI help in screening viral and COVID-19 pneumonia? IEEE 2020 132665-132676
Rasheed J, Hameed AA, Djeddi C, Jamil A, Al-Turjman F. A machine-learning based framework for diagnosis of COVID-19 from chest X-ray images.Interdiscip Sci Comput Life Sci 2021; 13, 103-117. doi:10.1007/s12539-020-00403-6.
El Asnaoui K Chawki Y. Using X-ray images and deep learning for automated detection of coronavirus disease. J Biomol Struct Dyn 2021; 39, 3615-3526 doi:10.1080/07391102.2020.1767212
Liang W, Yao J, Chen A, Lv Q, Zanin M, Liu J y col. Early triage of critically ill COVID-19 patients using deep learning. Nat Commun 2020, 11,3543-3550, doi:10,1038/s 41467-020-17280-8
Chen X, Tang Y, Mo Y, Li S, Lin D, Yang Z y col. A diagnostic model for coronavirus disease 2019 (COVID-19) based on radiological semantic and clinical features: a multi-center study. Eur Radiol 2020, 30, 4893-4902, doi:10.1007/s00330-020-06829-2.
Brat GA, Weber GM, Gehlenborg N, Avillach P, Palmer NP, Chivato L y col. International electronic health record-derived COVId-19 clinical course profiles: the 4CE consortium. Npj Digit Med 2020, 3,109-118. doi:10.1038/s41746-020-00208-0
Razavian N, Major VJ, Sudarshan M, Burk-Rafel J, Stella P, Randhawa H, y col. A validated real-time prediction model for favorable outcomes in hospitalized COVID-19 patients. Npj Digit Med 2020, 3, 130-143, doi:10.1038/s41746-020-00343-x
Yadaw AS, Li YC, Bose S, Iyengar R, Bunyavanich S, Pandey G. Clinical features of COVID-19 mortality: development and validation of a clinical prediction model. The Lancet Digital Health 2020, 2, e516-e525. doi:10.1016/S2589-7500(20)30217-X
Ji D, Zhang D, Xu J, Chen Z, Zang T, Zhao P y col. Prediction for progression risk in patients with COVID-19 pneumonia: the CALL score. Clin Infect Dis 2020, 71, 1393-1399, doi:10.1093/cid/ciaa414
Das JK, Chakraborty S, Roy S. A scheme for inferring viral-host associations based on codon usage patterns identifies the most affected signaling pathways during COVID-19. J Biomed Inform 2021, 118, 103801-103814, doi:10.10(6).jbi.2021.103801
Yaar E, Cola KC, Yologlu S. Artificial intelligence-based prediction of Covid-19 severity on the results of protein profiling. Comput Methods Programs Biomed 2021, 202, 105996-106007, doi:10.1016/j.cmpb.2021.105996
Wang J. Fast identification of possible drug treatment of coronavirus disease-19 (COVID-19) through computational drug repurposing study. J Chem Inf Model 2020, 60, (6), 3277-3296. doi:10.1021/acs.jcim.0c00179
Ton AT, Gentile F, Hsing M, Ban F, Cherkasov A. Rapid identification of potential inhibitors of SARS-CoV-2 main protease by deep docking of 1.3 billion compounds. Mol Inform 2020, 39, e2000028. doi:10.1002/minf.202000028.
Cavasotto CN, Di Filippo JI. In silico drug repurposing for COVID-19: targeting SARS-CoV-2 proteins through docking and consensus ranking. Mol Inform 2021 Jan;40(1):e2000115. doi: 10.1002/minf.202000115. Epub 2020 Aug 18.
Chen, B., Khodadoust, M. S., Olsson, N., Wagar, L. E., Fast, E., Liu, C. L., et al. Predicting HLA class II antigen presentation through integrated deep learning. Nat. Biotechnol 2019. 37, 1332–1343. doi: 10.1038/s41587-019-0280-2
Jurtz, V., Paul, S., Andreatta, M., Marcatili, P., Peters, B., and Nielsen, M. NetMHCpan-4.0: improved peptide–mhc class i interaction predictions integrating eluted ligand and peptide binding affinity data. J. Immunol 2017; 199, 3360–3368. doi: 10.4049/jimmunol.1700893
Fast, E., and Chen, B. Potential T-cell and B-cell Epitopes of 2019-nCoV. bioRxiv 2020 [Preprint]. doi: 10.1101/2020.02.19.955484 (texto sin revisión por pares)
Foppa LM, Kermack WO, McKendrick AG. “WO Kermack and AG McKendrick: A Seminal contribution to the mathematical theory of epidemics'' en “A Historical introduction to mathematical modeling of infectious diseases'' 2017, Editor IN Foppa, Cambridge, Massachusetts, US Academic Press, 59-87, doi:10.1016/b978-0-12-802260-3-00004-3.
Rahimi I, Chen F, Gandhi AH. A review on COVID-19 forecasting models. Neural computing and applications. Neural Comput Applic 2021, doi:10.1007/s00521-020-05626-8.
Ivorra B, Fernández MR, Vela-Pérez M Ramos AM. Mathematical modeling of the spread of the Coronavirus diseases 2019 (COVID-19). Taking into account the undetected infections. The case of China. Commun. Nonlinear Sci Numer Simulation 2020, 88, 105303-105355. doi:10.1016/j.cnsns.2020.105303
de Oliveira IS, Gruetzmacher SB, Teixeira JP. COVID-19 time series prediction. Proced Comput Sci 2021, 181, 973-980. doi:10.1016/j.procs.2021.01.254
Leslie L, Yeager MB. Balancing health privacy, health information exchange and re-search in the context of COVID-19 pandemic. J Am Med Inform Assoc 2020, 27, 963-966. doi:10.1093/jamia/ocaa039
Arun Kumar KE, Kalaga DV, Sai Kumar CM, Chilkoor G, Kawaji M, Brenza ™. Forecasting the dynamics of cumulative COVID-19 cases (confirmed, recovered and deaths) for top-16 countries using statistical machine learning models: auto-regressive integrated moving average (ARIMA) and seasonal auto-regressive integrated moving average (SARIMA). Appl Soft Comput 2021, 103, 107161-107187. doi:10.1016/j.asoc.2021.107161
Husnayain A, Fuad A, Su EC-Y. Applications of google search trends for risk communication in infectious disease management: a case study of COVID-19 outbreak in Taiwan. Int J Infect Dis. 2020;95:221-223.
Alimadadi A, Aryal S, Manandhar I, Munroe PB, Joe B, Cheng X. Artificial Intelligence and Machine Learning to Fight COVID-19. Physiol Genomics. 2020;52:200-202.
McCall B. COVID-19 and artificial intelligence: protecting health-care workers and curbing the spread. Lancet Digit Health. 2020;2:e166-e167.
Wosik J, Fudim M, Cameron B, Gellad ZF, Cho A, Phinney D, y col. Telehealth transformation: COVID and the rise of virtual care. J Am Med Inform Assoc 2020, 27, 957-962. doi:10.1093/jamia/ocaa067
Rao SR, Vázquez JA. Identification of COVID-19 can be quicker through artificial intelligence. Framework using a mobile phone-based survey when cities and towns are under quarantine. Infect Control Hosp Epidemiol 2020, 41, 826-830. doi:10.1017/ice.2020.61
Tucker A. Rapid implementation of a COVID-19 remote patient monitoring program. J Am Med Inform Assoc 2020, 27, 1326-1330. doi:10.1093/jamia/ocaa097.
Arshadi AK, Webb J, Salem M, Cruz E, Calad-Thompson S, Ghadirian N, Collins J, Díez-Cecilia E, Kelly B, Goodarzi H, Shiun Yuan J. Artificial intelligence for COVID-19 drug discovery and vaccine development. Front Artif Intell 2020, 3, 020 https://doi.org/10.3389/frai.2020.00065
Pizzo DT, Esteban S. IATos: study of an IA-powered pre-screening tool for COVID-19 from cough audio samples. https://arxiv.org/pdf/2104.13247.pdf (texto sin revisión por pares)
Stokes JM, Yang K, Swanson K, Jin W, Cubillos-Ruiz A, Donghia NM, MacNair CR, French S, Carfrae LA, Bloom-Ackermann Z, Tran VM, Chiappino-Pepe A, Badran AH, Andrews IW, Chory EJ, Church GM, Brown ED, Jaakola TS, Barzilay R, Collins JJ. A deep learning approach to antibiotic discovery. Cell 2020, 180, 688-702. doi:org/10.1016/j.cell.2020.01.021
Mayr A, Klambauer G, Unterthiner T, Steijaert M, Wegner J, Ceulemans H, Clevert DA, Hochreiter S. Large-scale comparison of machine learning methods for drug target prediction on ChEMBL. Chem Sci 2018, 9, 5441-5451
Wu Z, Ramsundar B, Feinberg EN, Gomes J, Geniesse C, Pappu AS, Leswing K, Pande V. MoleculeNet: a benchmark for molecular machine learning. Chem Sci 2017, 9, 513-530.
Yang K, Swanson K, Jin W, Coley C, Eiden P, Gao H, Guzmán-Pérez A, Hopper T, Kelley H, Mathea M, Palmer A, Settels V, Jaakkola T, Jensen K, Barzilay R. Analyzing learned molecular representations for property prediction. J Chem Inf Model 2019. 13:10.1021/acs/jcim.9b00237.
Brown DG, May-Dracka TL, Gagnon MM, Tommasi R, trends and exceptions of physical properties on antibacterial activity from Gram-positive and Gram-negative pathogens. J Med Chem 2014, 57, 10144-10161
De SK, Stebbins JL, Chen LH, Riel-Mehan M, Machleidt T, Dahl R, Yuan H, Emdadi A, Barile E, Chen V Murphy R, Pellecchia M. Design, synthesis and structure-activity relationship of substrate competitive, selective and in vivo active triazole and thiadiazole inhibitors of the c-Jun N-terminal kinase. J Med Chem 2009, 52, 1943-1952.
Jang S, Yu LR, Abdelmegeed MA, Gao Y, Banerjee A, Song BJ. Critical role of c-jun N-terminal protein kinase in promoting mitochondrial dysfunction and acute liver injury. Redox Biol 2015, 6, 552-564.
Tommasi R, Brown DG, Walkup GK, Manchester JL, Miller AA, ESKAPEing the labyrinth of antibacterial discovery Mat Rev Drug Discov 2015, 14, 529-542.
Stokes JM, Brown ED. Chemical modulators of ribosome biogenesis as biological probes. Nat Chem Biol 2015, 11, 924-032.
Haks MC, Bottazzi B, Cecchinato V, y col. Molecular signatures of immunity and immunogenicity in infection and vaccination. Front Immunol 2017;8. doi:10.3389/fimmu.2017.01563
Lundegaard C, Lund O, Nielsen M. Prediction of epitopes using neural network based methods. J Immunol Methods 2011;374(1-2):26-34.
Moxon R, Reche PA, Rappuoli R. Editorial: reverse vaccinology. Front Immunol 2019; 10:1-2
Mak KK, Pichika MR. Artificial intelligence in drug development: present status and future prospects. Drug Discov Today 2019;24(3):773-780
Pappalardo F, Flower D, Russo G, y col. Computational modeling approaches to vaccinology. Pharmacol Res (internet) 2015;92:40-45.
Russo G, Reche P, Pennisi M, Pappalardo F. The combination of artificial intelligence and systems biology for intelligent vaccine design. Exp Opin Drug Discov 2020; doi:10.1080/17460441.2020.1791076
De Groot AS, Bosma A, Chinai N, y col. From genome to vaccine: in silico predictions, ex vivo verification. Vaccine 2001;19(3):4385-4395.
Christensen DM, Manley J, Resendez J. Medical algorithms are failing communities of color. Health Affairs blog. Sep 9, 2021. https://www.healthaffairs.org/do/10.1377/forefront.20210903.976632/ (Acceso 5 Abr 2023)
Olsson, H., Kartasalo, K., Mulliqi, N. et al. Estimating diagnostic uncertainty in artificial intelligence assisted pathology using conformal prediction. Nat Commun 13, 7761 (2022). https://doi.org/10.1038/s41467-022-34945-8
Embi PJ. Algorithmovigilance - Advancing methods to analyze and monitor artificial intelligence-driven health care for effectiveness and equity. JAMA Network Open 2021; 4(4):e214622. doi:10.1001/jama network open.2021.4622
US Food and Drug Administration. Artificial intelligence and machine learning in software as a medical device. https://www.fda.gov/medical-devices/software-medical-device-samd/artificial-intelligence-and-machine-learning-software-medical-device (Acceso 5 Abr 2023)
Beam AL, Drazen JM, Kohane IS, Leong T-Y. Manrai AK, Rubin EJ. Artificial intelligence in medicine. N Engl J Med 2023; 388(13):1220-21.
Dorr DA, Adams L, Embi P. Harnessing the promise of artificial intelligence responsibly. JAMA 2023; doi:10.1001/jama.2023.2771
Harari Y, Harris T, Razkin A. You can have the blue pill or the red pill, and we´re out of blue pills. New York Times, Guest Essay, Marzo 24, 2023.
Jiao Z, Ji H, Yan J, Qi X. Application of big data and artificial intelligence in epidemic surveillance and containment. Intelligent Med 2023 (3); 36-43.
Kuhn TS. The structure of scientific revolutions. Chicago. University of Chicago Press 1970. ISBN 0-226-45803-2
Belloso WH. On innovation. Ther Innov Reg Science 2020,Feb 5. https://doi.org/10.1007/s43441-020-00125-3
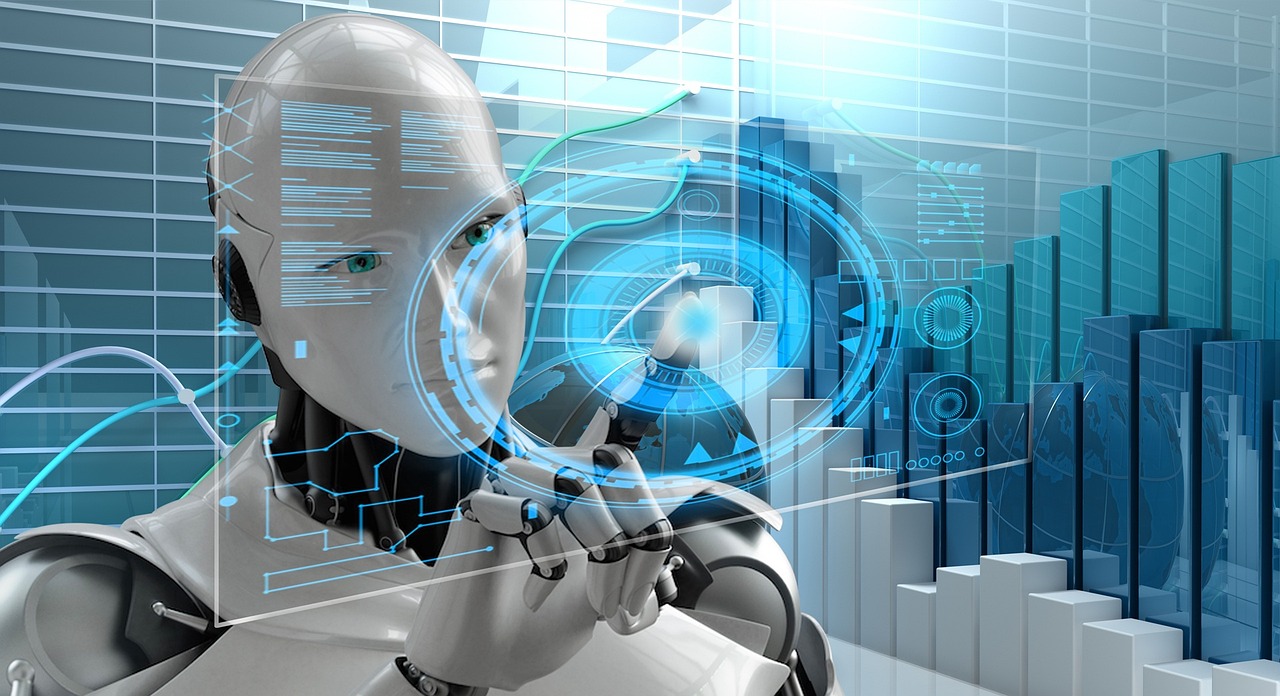